- Home
- Faculty
- School of Computer Science
- Dr. Amit Ranjan
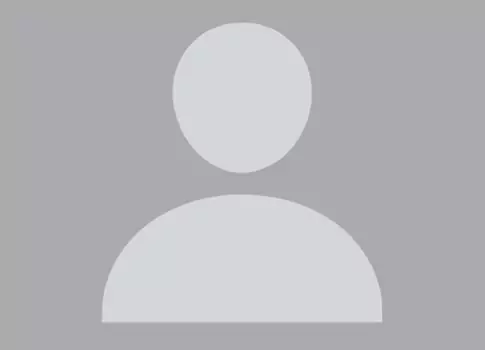
Dr. Amit Ranjan
Assistant Professor
Profile Summary
Dr. Amit Ranjan is a dedicated researcher and academician specializing in Machine Learning, Deep Learning, Graph Neural Networks, and Drug Discovery. He holds a Ph.D. in Computer Science and Engineering from the Indian Institute of Technology Patna, where his research focused on deep generative models for drug discovery and medical image synthesis. His work integrates artificial intelligence with biomedical applications, particularly in predicting drug-target interactions and antibiotic resistance genes. Prior to joining UPES, Dr. Ranjan was a Postdoctoral Researcher at Louisiana State University, USA, where he contributed to cutting-edge projects on AI-driven drug discovery and environmental antibiotic resistance prediction.
Work Experience
- Assistant Professor, School of Computer Science, UPES, Dehradun (Current)
- Postdoctoral Researcher, Louisiana State University, Baton Rouge, LA, USA (Sept 2023 - Current)
- Research Associate 2, Louisiana State University, Baton Rouge, LA, USA (Jan 2023 - Sept 2023)
Research Interests
Dr. Amit Ranjan's research focuses on applying AI and deep learning to biomedical challenges. His key interests include:
- Drug discovery using deep generative models
- Antibiotic resistance gene prediction
- Graph Neural Networks for biomedical applications
- Reinforcement learning for molecule generation
- Machine learning for medical imaging analysis
- Large-scale biological data analysis
His research aims to bridge the gap between computational modeling and real-world biomedical applications, ensuring AI-driven solutions for healthcare challenges.
Teaching Philosophy
Dr. Ranjan believes in a hands-on, research-driven approach to teaching. He emphasizes:
- Application-based learning using real-world datasets
- Encouraging interdisciplinary collaboration between AI and biomedical sciences
- Use of open-source tools like Python, TensorFlow, and PyTorch for practical implementation
- Bridging the gap between theoretical learning and industry-oriented skills
Courses Taught
- Machine Learning and Deep Learning
- Big Data Computing
- Reinforcement Learning
- Distributed Systems and Algorithms
- Graph Neural Networks and their Applications
Awards and Grants
- Microsoft Azure AI for Health COVID-19 Grant (2020)
- Best Paper Award at International Conference on Artificial Intelligence and Evolutionary Computations in Engineering Systems (ICAIECES 2019)
- NPTEL Teaching Assistance Certification (2023)
- Google Cloud Training Certification on Reinforcement Learning (2021)
- GIAN Certification on Distributed Systems & Machine Learning (2022)
Scholarly Activities
- Published impactful research in Journal of Chemical Information and Modeling, Biomedical Optics Express, and IEEE Transactions
- Presented research at ICML, IEEE Big Data, and AI-ML Systems conferences
- Reviewer for IEEE Transactions on Artificial Intelligence, BioData Mining, and Knowledge-Based Systems
- Developed open-source AI tools for drug discovery and resistance gene prediction
- Mentored students in research projects related to AI in biomedical sciences
- Organized workshops on Graph Neural Networks and AI for Drug Discovery
Key Publications
- MDF-DTA: A Multi-Dimensional Fusion Approach for Drug-Target Binding Affinity Prediction - Journal of Chemical Information and Modeling, 2024.
- KG-DTA: A knowledge graph-based meta-path learning framework for predicting drug-target binding affinity - IEEE AI-ML Systems, 2024.
- Trans-ARG: Predicting Antibiotic Resistance Genes with a Transformer-Based Model - IEEE AI-ML Systems, 2024.
- GNN-ARG: A Graph Neural Network-based Framework for Predicting Antibiotic Resistance Genes - IEEE Big Data, 2024.
Contact