Unlocking the Potential: Machine Learning’s Impact on Computer Science and Engineering
- Dr. Ravi S. Iyer
- Published 25/09/2023
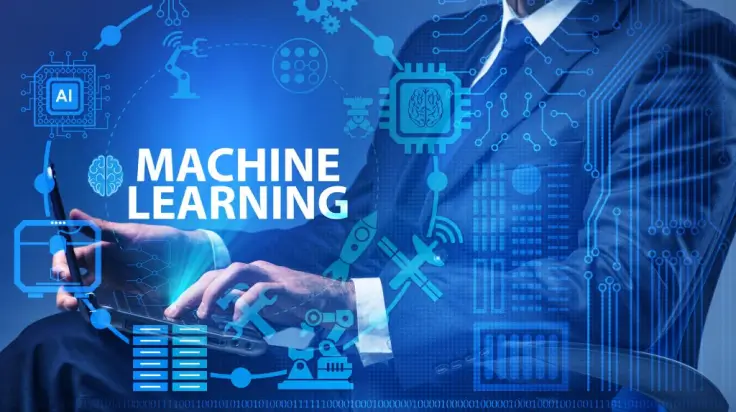
One technology that has evolved continuously and found widespread application and acceptance in every conceivable part of life is Machine Learning (ML). Not only has this field altered the way we approach problem-solving, but it has also unlocked opportunities in many fields that were previously unimaginable. The future of computer science and engineering is being significantly influenced by machine learning.
Machine Learning (ML) is a subset of artificial intelligence that focuses on the development of algorithms and statistical models, allowing computer systems to learn and make predictions or decisions without explicit programming. It leverages the power of data and computational capabilities to analyse patterns, make predictions, and drive intelligent decision-making.
The applicability of ML to various applications spanning computer science and engineering is one of its most notable features. ML algorithms have facilitated advancements in image identification, object detection, and facial recognition in computer vision. The now ubiquitous ChatGPT and its ilk, sentiment analysis tools, and language translation have all been made possible by ML in the field of Natural Language Processing (NLP). The addition of ML to cybersecurity has improved network security and threat identification. These programs have changed how we connect with technology and made space for fresh opportunities.
ML has rekindled research in the field of computer science including speech recognition, machine translation, and recommendation systems. In many areas, techniques like deep learning neural networks have demonstrated amazing performance, often exceeding human capabilities. Way back, in 2014, Jeremy Howard, in his Ted talk titled “The wonderful and terrifying implications of computers that can learn”, referred to an ML tool capable of translating in real time from English to Mandarin. Technology has in the intervening decade grown leaps and bounds, and such tools have far exceeded the capabilities of a human interpreter. Large Language Models have considerably increased machine translation systems’ accuracy, making them indispensable for international communication.
Another significant contribution to the world has been Reinforcement Learning, an ML technique. It allows machines to learn through a mechanism of rewards and penalties. Agents can learn by interacting with their environment and receiving feedback. This paradigm has sparked advancements in robotics and autonomous systems, such as self-driving vehicles, drones, and robots that can carry out intricate tasks precisely while learning from their environment. It is also used in algorithmic trading to develop strategies that can make automated decisions on buying and selling financial instruments.
Machine learning has ushered in dramatic advances in the engineering profession. Devices connected to the Internet of Things (IoT) are now smarter and more productive thanks to the incorporation of ML algorithms. For instance, ML is used by smart home systems to optimise energy utilisation and improve sustainability. The predictive skills of ML are essential for industrial machinery predictive maintenance, assisting in reducing downtime and increasing productivity.
Moreover, ML plays a pivotal role in data analysis, a critical component of engineering projects. Engineers now use ML to process massive datasets generated by sensors and simulations. ML algorithms can quickly identify patterns and anomalies, assisting engineers in making informed decisions and optimising designs.
The way engineers develop structures and products using computer-aided design (CAD) is changing thanks to generative algorithms driven by machine learning (ML). These algorithms generate a large number of design possibilities based on user-defined parameters, accelerating the design process, and producing more creative and effective results.
However, with great power comes great responsibility. The widespread adoption of ML also raises ethical and societal concerns. Bias in ML models, privacy violations, and job displacement are significant challenges that need to be addressed. Computer scientists and engineers must work hand-in-hand to develop ethical guidelines, ensure transparency, and minimise the negative impacts of ML technologies.
In conclusion, machine learning has a profound and wide-ranging influence on computer science and engineering. It has transformed problem-solving, opened up new avenues for opportunity, and simplified previously challenging activities. Intelligent systems, better decision-making, and quicker procedures have all been made possible by the integration of ML into computer science and engineering. It is essential to carefully consider the ethical and societal ramifications as we continue to realise the potential of machine learning.
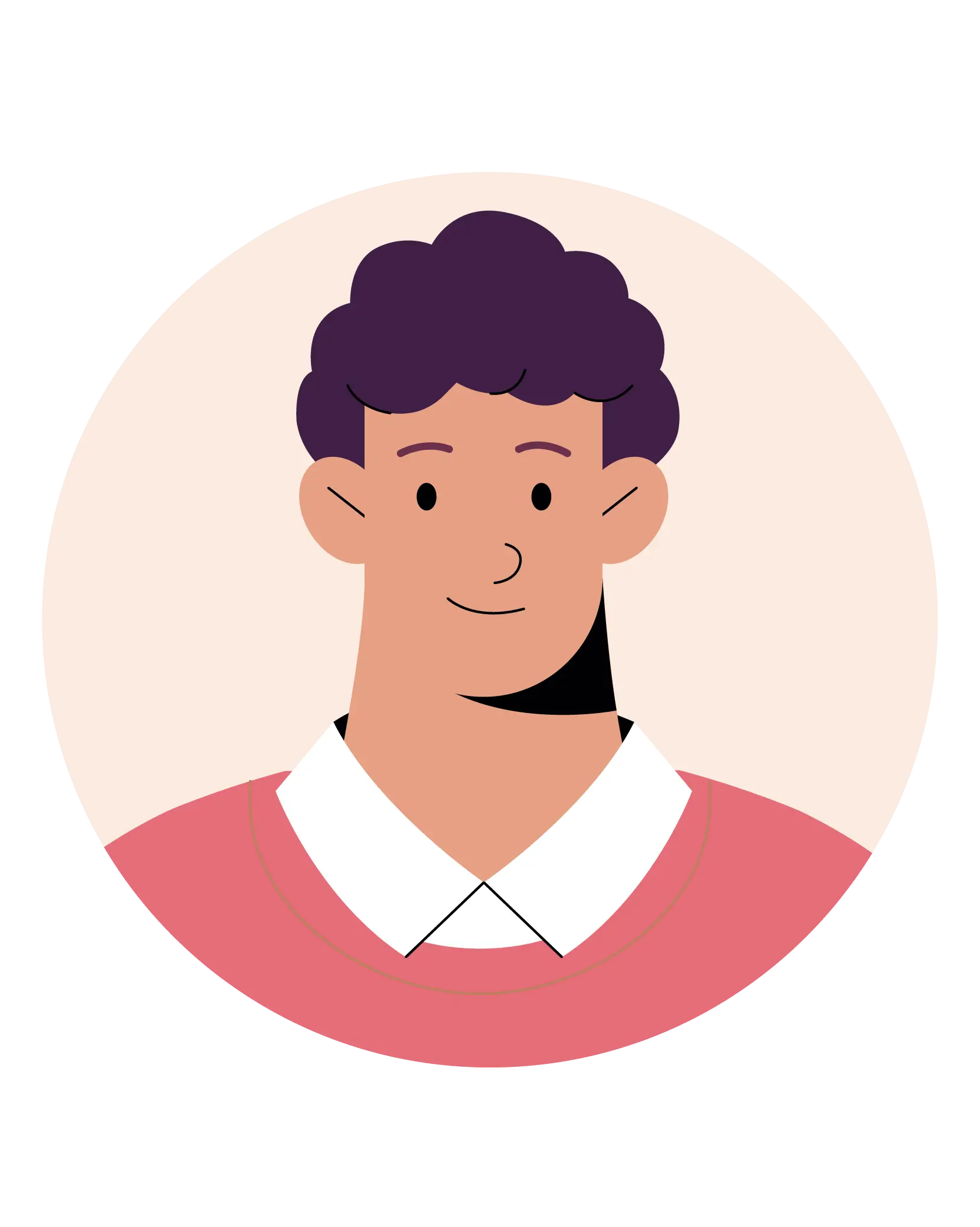
Dr. Ravi S. Iyer
The writer is Dr. Ravi S. Iyer, Dean, UPES School of Computer Science
UPES Admission Enquiry
Subscribe to UPES Blogs
Join our community for exclusive stories, insights, and updates
By clicking the "Subscribe" button, I agree and accept the privacy policy of UPES.